Merging nucleus datasets by correlation-based cross-training |
Wenhua Zhang*1,2, Jun Zhang*2, Sen Yang2, Xiyue Wang2, Wei Yang2, Junzhou Huang2, Wenping Wang#1,3, Xiao Han#2 |
1The University of Hong Kong, 2Tencent AI Lab, 3Texas A&M University, |
|
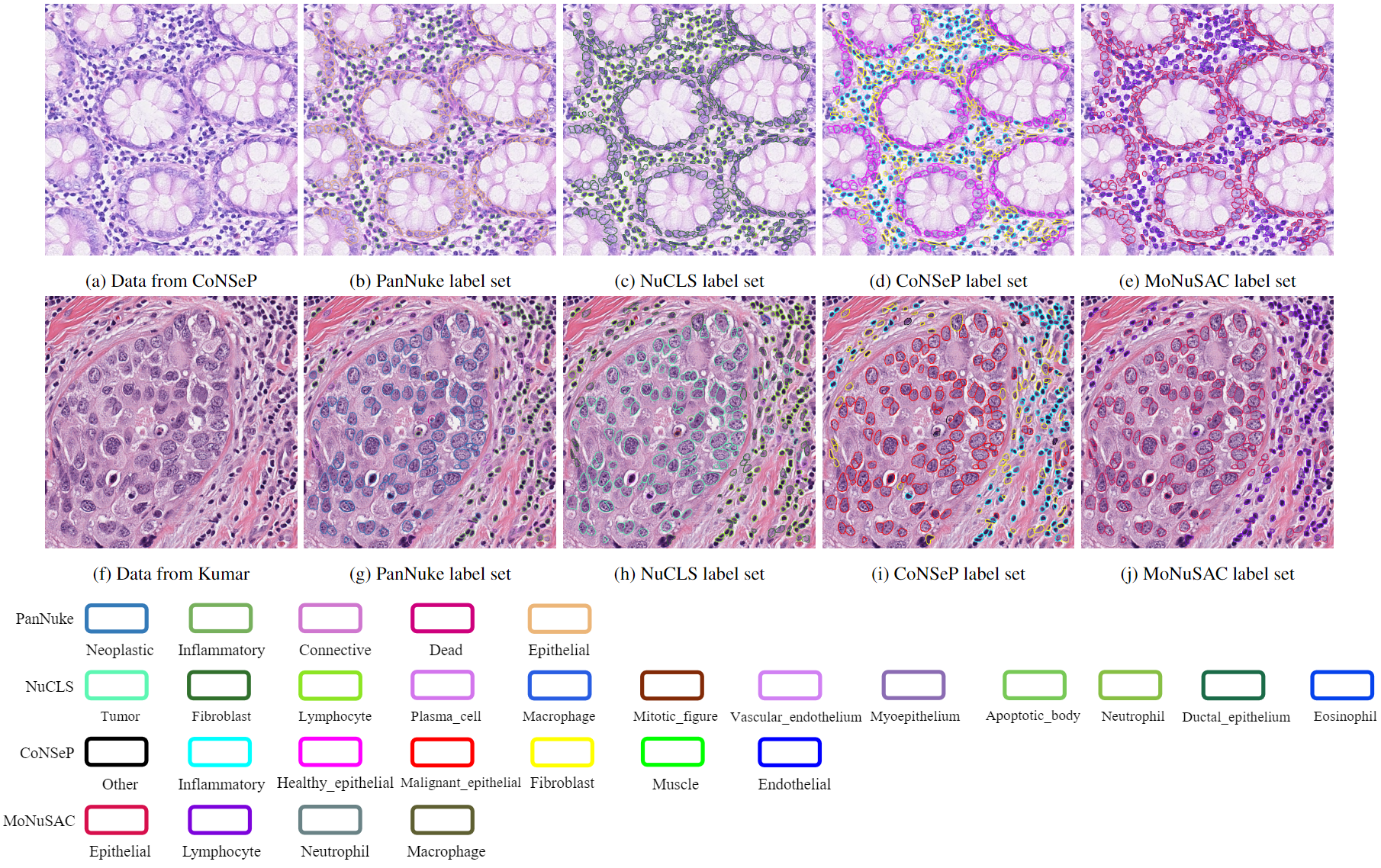 |
Fig. 1. Example of visual results from the merged dataset. The top row shows an image from the CoNSeP dataset and its four corresponding classifications in the merged dataset. It has one ground-truth labeling (The CoNSeP label set) and three predicted classifications. The bottom row shows an image from the Kumar dataset with its four corresponding classifications all predicted by our framework.
|
Data |
|
Data format |
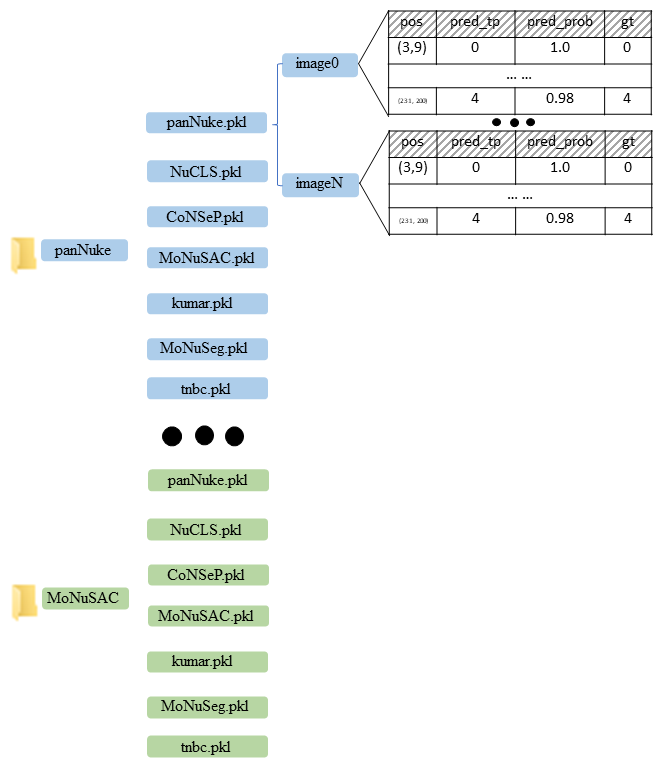 |
|
We organize the output of our algorithm into a final merged dataset. For each label set (panNuke, NuCLS, CoNSeP, MoNuSAC), Wwe show the data from all datasets (panNuke, NuCLS, CoNSeP, MoNuSAC, kumar, MoNuSeg, TNBC) that we used and our prediction for each nucleus. We use a pickle file for each dataset under each label set. Each pickle file is a dictionary whose keys are the image filenames, and the contents are the instance predictions. For each prediction, the first item is the location of the nuclear centroid, the second is our predicted classification label, the third is the probability of the predicted label, and the last is the ground-truth label, if available. We set it as -1 if there is no ground-truth label for the nucleus under the label set.
We also include overlay images with different colors for different classification labels for visualization. The code to draw the overlay images is also released to demonstrate the data usage.
|
|
Code |
|
Abstract |
Fine-grained nucleus classification is challenging because of the high inter-class similarity and intra-class variability. Therefore, a large number of labeled data is required for training effective nucleus classification models. However, it is challenging to label a large-scale nucleus classification dataset comparable to ImageNet in natural images, considering that high-quality nucleus labeling requires specific domain knowledge. In addition, the existing publicly available datasets are often inconsistently labeled with divergent labeling criteria. Due to this inconsistency, conventional models have to be trained on each dataset separately and work independently to infer their own classification results, limiting their classification performance. To fully utilize all annotated datasets, we formulate the nucleus classification task as a multi-label problem with missing labels to utilize all datasets in a unified framework. Specifically, we merge all datasets and combine their labels as multiple labels. Thus, each data has one ground-truth label and several missing labels. We devise a base classification module which is trained using all data but sparsely supervised by the ground-truth labels only. We then exploit the correlation among different label sets by a label correlation module. By doing so, we can have two trained basic modules and further cross-train them with both ground-truth labels and pseudo labels for the missing ones. Importantly, data without any ground-truth labels can also be involved in our framework, as we can regard them as data with all labels missing and generate the corresponding pseudo labels. We carefully re-organized multiple publicly available nucleus classification datasets, converted them into a uniform format, and tested the proposed framework on them. Experimental results show substantial improvement compared to the state-of-the-art methods. |
|
Network Overview |
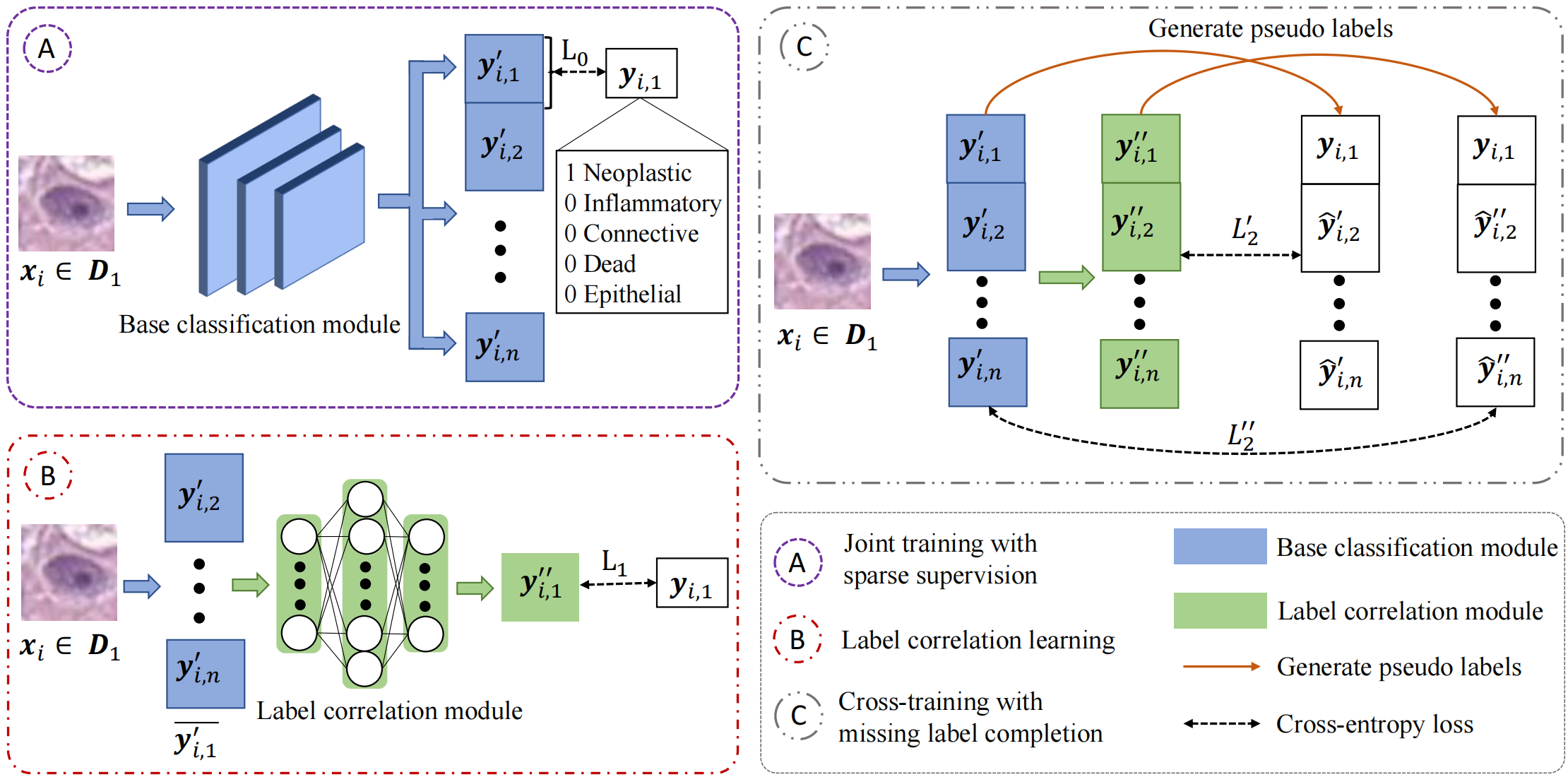 |
Fig. 2.Framework of our method. We use data xi from D1 as an example here. Our pipeline consists of three main training steps: A) joint training with sparse supervision, B) label correlation learning, and C) cross-training with label completion. We first train the base classification module and label correlation module individually with ground truth labels yi,1 with cross entropy cosses L0 and L1 (Step A and B). We then cross-train the two modules with both the ground truth labels yi,1 and the mutually completed pseudo-labels(Step C).
|
|
©Wenhua Zhang. Last update: Nov., 2022. |